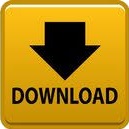
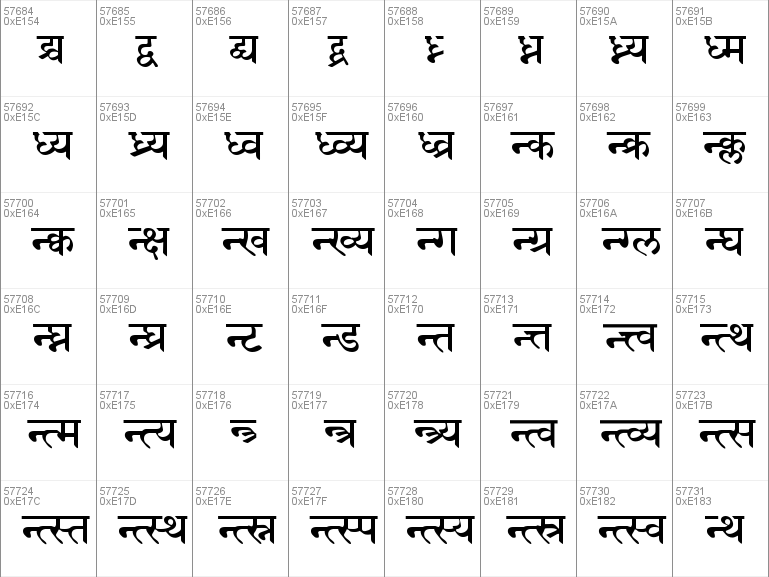
In this paper, we show that PG can also be applied to English resulting in an elegant computational grammar. Published in South Asian Language Review, Creative Books, New Delhi, 1998.] Computational Paninian Grammar framework (PG) has been successfully applied to modern Indian languages earlier, using which anusaaraka machine translation system has been built (Narayana, 1994). In all the tasks we discuss for Sanskrit, we either achieve state of the art results or ours is the only data driven solution for those tasks. We obtain significant improvements in morphosyntactic tasks for Sanskrit by incorporating language specific constraints into the model. Moreover, our framework enables to incorporate language specific constraints to prune the search space and to filter the candidates during inference. Our experiments in Czech and Sanskrit show the language agnostic nature of the framework, where we train highly competitive models for both the languages.
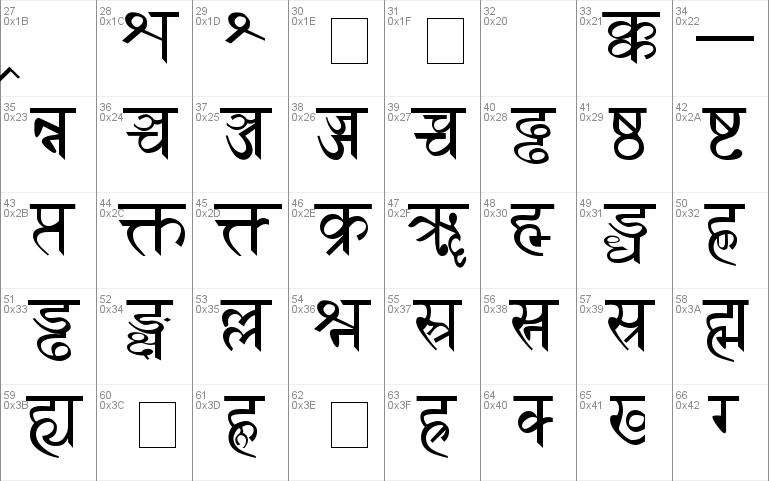
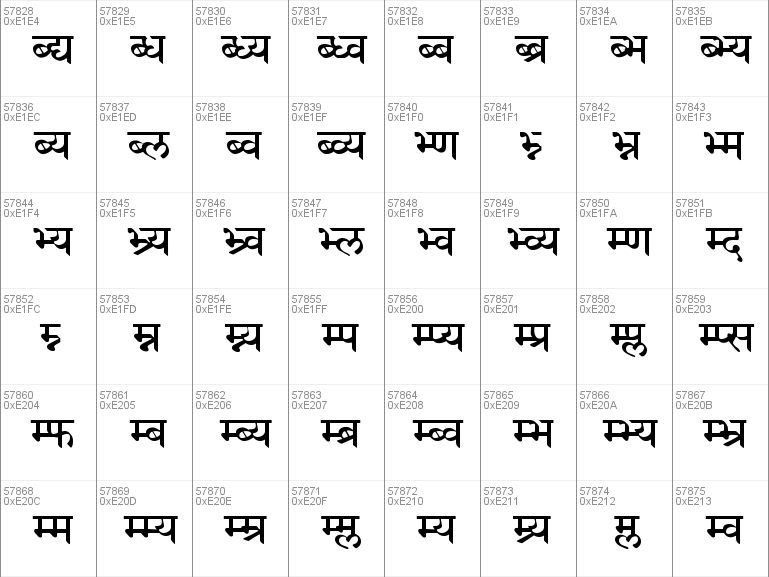
This enables us to substantially reduce the training data requirements to as low as 10 % as compared to the data requirements for the neural state of the art models.
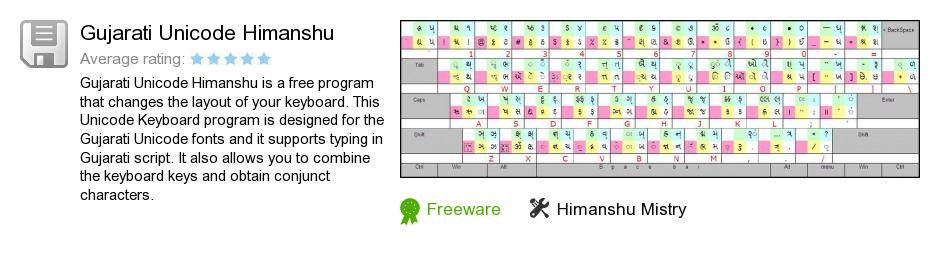
The feature function so learnt along with the search space we construct, encode relevant linguistic information for the tasks we consider. But here, we automate the learning of the feature function. Typically the state of the art models for morphosyntactic tasks in morphologically rich languages still rely on hand-crafted features for their performance. Ours is a search based structured prediction framework, which expects a graph as input, where relevant linguistic information is encoded in the nodes, and the edges are then used to indicate the association between these nodes. Ours is an arc-factored model, similar to the graph based parsing approaches, and we consider the tasks of word-segmentation, morphological parsing, dependency parsing, syntactic linearisation and prosodification, a prosody level task we introduce in this work. We propose a framework using Energy Based Models for multiple structured prediction tasks in Sanskrit. We also investigate the impact of word ordering in which the sentences are provided as input to these systems, by parsing verses and their corresponding prose order (anvaya) sentences. In this work, we analyse the performance of the parsers using both an in-domain and an out-of-domain test dataset. Further, since our focus is on the learning power of each of the models, we do not incorporate any Sanskrit specific features explicitly into the models, and rather use the default settings in each of the paper for obtaining the feature functions. We compare the performance of each of the models in a low-resource setting, with 1,500 sentences for training. We experiment with 2 graph based and 2 transition based parsers. In this work, we evaluate four different data-driven machine learning models, originally proposed for different languages, and compare their performances on Sanskrit data. This can primarily be attributed to the lack of availability of task-specific labelled data and the morphologically rich nature of the language. However, Sanskrit still lacks a robust purely data-driven dependency parser, probably with an exception to Krishna (2019). Data-driven approaches for dependency parsing have been of great interest in Natural Language Processing for the past couple of decades.
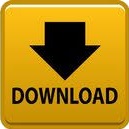